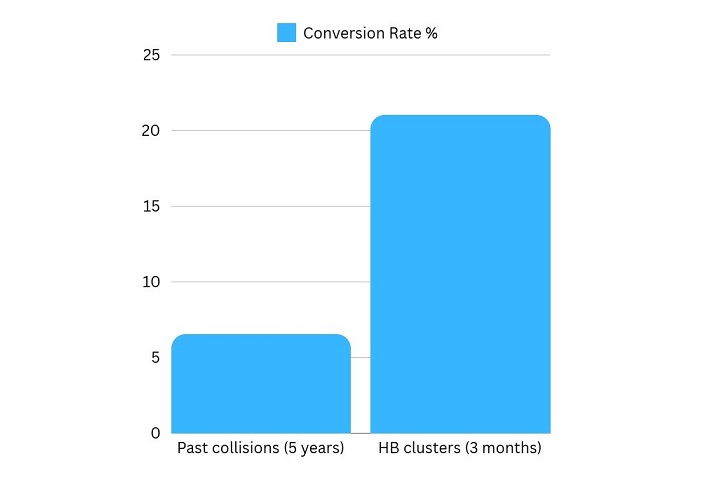
A new study has found that connected vehicle data is more effective at predicting road collisions than traditional analysis of historic datasets.
The study, carried out by Aisin RoadTrace, compared the predictive efficiency of two methods:
- The traditional approach, which considers the locations of historic collisions as the most dangerous hotspots of the road network.
- An approach which considers the clusters of harsh braking events as future collision location candidates.
The aim was to determine whether future crashes can be predicted more accurately.
To conduct the analysis, the researchers used a set of data from connected vehicles driving over a large-scale road network in southeast England.
This included two million harsh braking events – defined as the threshold at which all the objects in a car that are not well stowed would fly off the seat.
In addition to this, they used a dataset of six years of validated collision reports.
The results indicate that harsh braking events bring comparatively more information than past collisions.
Clusters of harsh braking bring an improvement of 22% in prediction rate compared to an equivalent number of historical collision locations.
Additionally, researchers say they have shown that using harsh braking clusters allows a much quicker detection of future collisions – as well as having the ability to detect collisions that could not be predicted even using five years of historical road collision data.
The researchers say the data represents an untapped data mine for road engineers who oversee road quality and safety.
Those interested in hearing more about the study can attend a free webinar, taking place on 10 October. Click here to register.
Worked with local bus company operations using their telematics on emergency braking to see if could determine if a pedestrian step out campaign had any effect in real life a few years ago. Used specific route which has buses every 10 mins where campaign ran on digital bus stops etc. Did before, during and month after basic analysis. Looked promising but didn’t have resource to continue or test robustly. Would be interesting to do this properly. Anyone else tried something similar ?
Keith Baldock, Brighton
0
Sounds like a (welcome) extension to Brian Spicer’s work, featured in the Cardington course in the early ’80s.
e.g. Older, S. J., & Spicer, B. R. (1976). Traffic Conflicts—A Development in Accident Research. Human Factors, 18(4), 335-350. https://doi.org/10.1177/001872087601800403
Of course, these were in the days when we referred to road traffic accidents by their proper name. 🙂
Fraser Andrew, STIRLING
0